Visualizing Temporal Topic Embeddings with a Compass
Daniel Palamarchuk - Virginia Tech, Blacksburg, United States
Lemara Williams - Virginia Polytechnic Institute of Technology , Blacksburg, United States
Brian Mayer - Virginia Tech, Blacksburg, United States
Thomas Danielson - Savannah River National Laboratory, Aiken, United States
Rebecca Faust - Tulane University, New Orleans, United States
Larry M Deschaine PhD - Savannah River National Laboratory, Aiken, United States
Chris North - Virginia Tech, Blacksburg, United States
Download Supplemental Material
Room: Bayshore I
2024-10-17T12:30:00ZGMT-0600Change your timezone on the schedule page
2024-10-17T12:30:00Z
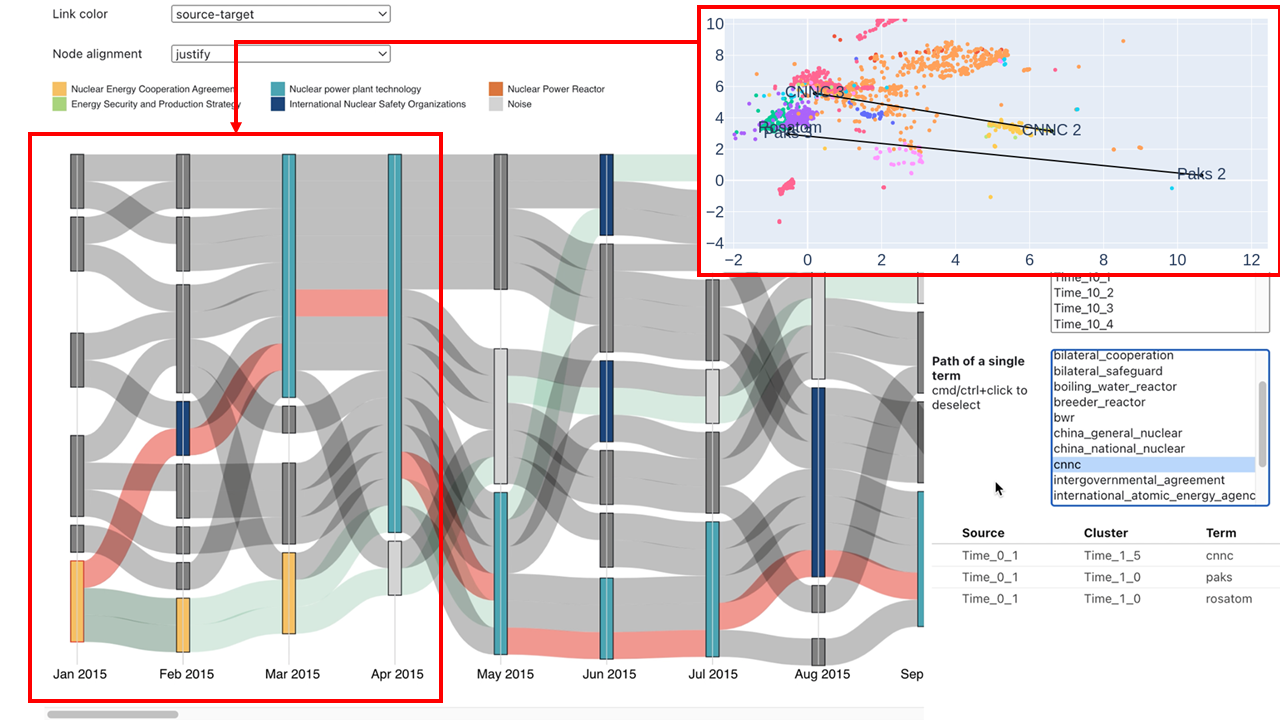
Fast forward
Full Video
Keywords
High dimensional data, Dynamic topic modeling, Cluster analysis
Abstract
Dynamic topic modeling is useful at discovering the development and change in latent topics over time. However, present methodology relies on algorithms that separate document and word representations. This prevents the creation of a meaningful embedding space where changes in word usage and documents can be directly analyzed in a temporal context. This paper proposes an expansion of the compass-aligned temporal Word2Vec methodology into dynamic topic modeling. Such a method allows for the direct comparison of word and document embeddings across time in dynamic topics. This enables the creation of visualizations that incorporate temporal word embeddings within the context of documents into topic visualizations. In experiments against the current state-of-the-art, our proposed method demonstrates overall competitive performance in topic relevancy and diversity across temporal datasets of varying size. Simultaneously, it provides insightful visualizations focused on temporal word embeddings while maintaining the insights provided by global topic evolution, advancing our understanding of how topics evolve over time.