Diffusion Explainer: Visual Explanation for Text-to-image Stable Diffusion
Seongmin Lee - Georgia Tech, Atlanta, United States
Benjamin Hoover - GA Tech, Atlanta, United States. IBM Research AI, Cambridge, United States
Hendrik Strobelt - IBM Research AI, Cambridge, United States
Zijie J. Wang - Georgia Tech, Atlanta, United States
ShengYun Peng - Georgia Institute of Technology, Atlanta, United States
Austin P Wright - Georgia Institute of Technology , Atlanta , United States
Kevin Li - Georgia Institute of Technology, Atlanta, United States
Haekyu Park - Georgia Institute of Technology, Atlanta, United States
Haoyang Yang - Georgia Institute of Technology, Atlanta, United States
Duen Horng (Polo) Chau - Georgia Tech, Atlanta, United States
Screen-reader Accessible PDF
Download preprint PDF
Room: Bayshore VI
2024-10-17T17:54:00ZGMT-0600Change your timezone on the schedule page
2024-10-17T17:54:00Z
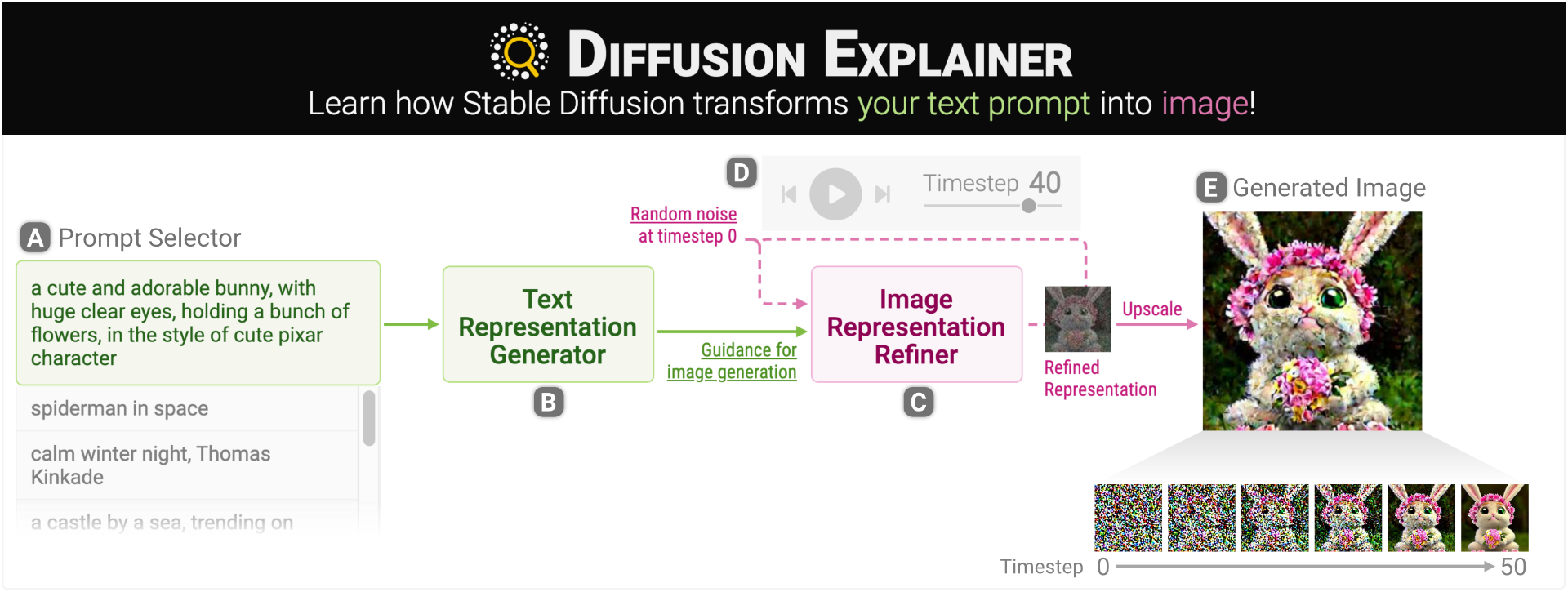
Fast forward
Full Video
Keywords
Machine Learning, Statistics, Modelling, and Simulation Applications; Software Prototype
Abstract
Diffusion-based generative models’ impressive ability to create convincing images has garnered global attention. However, their complex structures and operations often pose challenges for non-experts to grasp. We present Diffusion Explainer, the first interactive visualization tool that explains how Stable Diffusion transforms text prompts into images. Diffusion Explainer tightly integrates a visual overview of Stable Diffusion’s complex structure with explanations of the underlying operations. By comparing image generation of prompt variants, users can discover the impact of keyword changes on image generation. A 56-participant user study demonstrates that Diffusion Explainer offers substantial learning benefits to non-experts. Our tool has been used by over 10,300 users from 124 countries at https://poloclub.github.io/diffusion-explainer/.