Steering LLM Summarization with Visual Workspaces for Sensemaking
Xuxin Tang - Computer Science Department, Blacksburg, United States
Eric Krokos - Dod, Laurel, United States
Kirsten Whitley - Department of Defense, College Park, United States
Can Liu - City University of Hong Kong, Hong Kong, China
Naren Ramakrishnan - Virginia Tech, Blacksburg, United States
Chris North - Virginia Tech, Blacksburg, United States
Room: Bayshore II
2024-10-14T16:00:00ZGMT-0600Change your timezone on the schedule page
2024-10-14T16:00:00Z
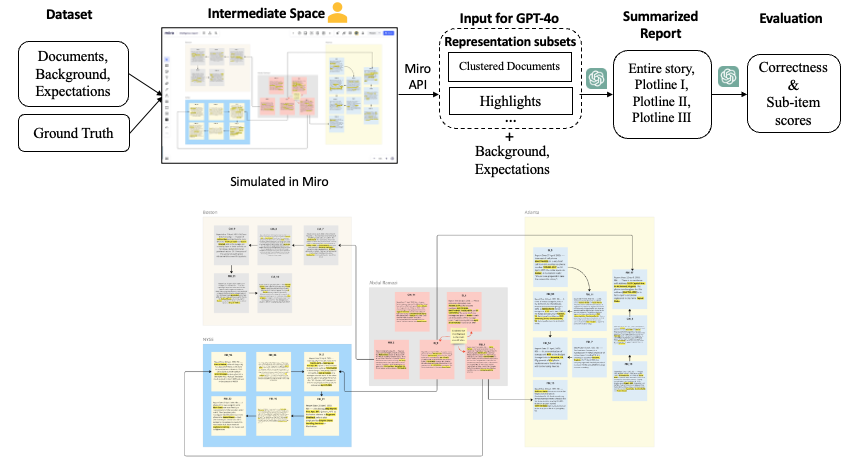
Abstract
Large Language Models (LLMs) have been widely applied in summarization due to their speedy and high-quality text generation. Summarization for sensemaking involves information compression and insight extraction. Human guidance in sensemaking tasks can prioritize and cluster relevant information for LLMs. However, users must translate their cognitive thinking into natural language to communicate with LLMs. Can we use more readable and operable visual representations to guide the summarization process for sensemaking? Therefore, we propose introducing an intermediate step--a schematic visual workspace for human sensemaking--before the LLM generation to steer and refine the summarization process. We conduct a series of proof-of-concept experiments to investigate the potential for enhancing the summarization by GPT-4 through visual workspaces. Leveraging a textual sensemaking dataset with a ground truth summary, we evaluate the impact of a human-generated visual workspace on LLM-generated summarization of the dataset and assess the effectiveness of space-steered summarization. We categorize several types of extractable information from typical human workspaces that can be injected into engineered prompts to steer the LLM summarization. The results demonstrate how such workspaces can help align an LLM with the ground truth, leading to more accurate summarization results than without the workspaces.