Visualizing Spatial Semantics of Dimensionally Reduced Text Embeddings
Wei Liu - Computer Science, Virginia Tech, Blacksburg, United States
Chris North - Virginia Tech, Blacksburg, United States
Rebecca Faust - Tulane University, New Orleans, United States
Download preprint PDF
Room: Bayshore II
2024-10-14T16:00:00ZGMT-0600Change your timezone on the schedule page
2024-10-14T16:00:00Z
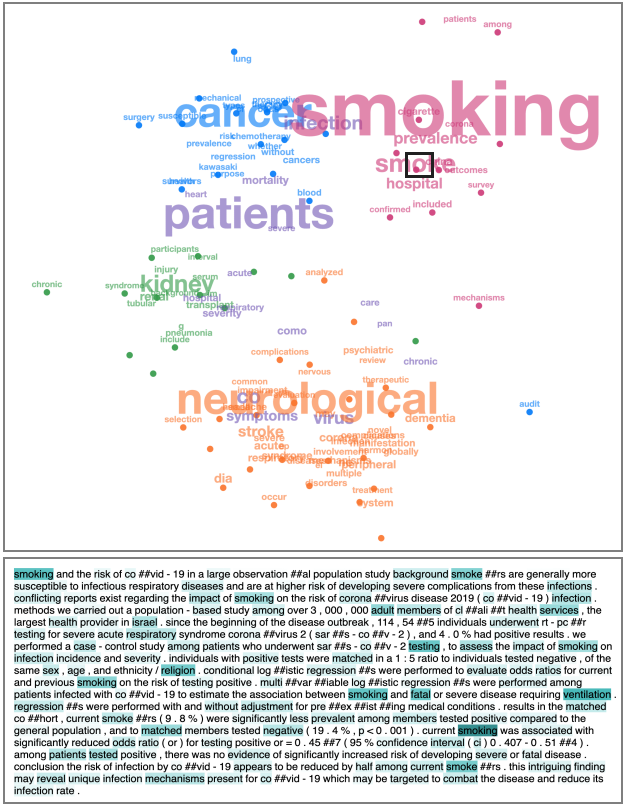
Fast forward
Abstract
Dimension reduction (DR) can transform high-dimensional text embeddings into a 2D visual projection facilitating the exploration of document similarities. However, the projection often lacks connection to the text semantics, due to the opaque nature of text embeddings and non-linear dimension reductions. To address these problems, we propose a gradient-based method for visualizing the spatial semantics of dimensionally reduced text embeddings. This method employs gradients to assess the sensitivity of the projected documents with respect to the underlying words. The method can be applied to existing DR algorithms and text embedding models. Using these gradients, we designed a visualization system that incorporates spatial word clouds into the document projection space to illustrate the impactful text features. We further present three usage scenarios that demonstrate the practical applications of our system to facilitate the discovery and interpretation of underlying semantics in text projections.